Learning Common Sense via Visual Abstractions
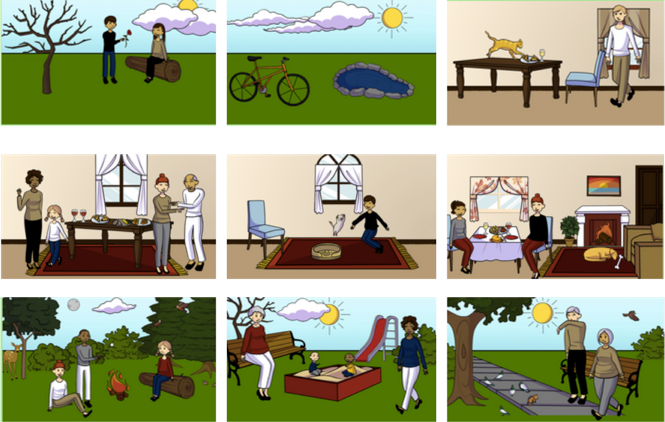
| September 1, 2014
Learning Common Sense via Visual Abstractions leverages abstract scenes to teach machines common sense.
Machines today can perform certain sophisticated but niche tasks quite well (e.g. play chess, play Jeopardy, vacuum our floors, drive autonomously). However, they are far from being sapient intelligent entities.
This is partly because they lack “common sense” knowledge — basic knowledge like birds fly, children are afraid of bears, kicking a ball makes it move. This common sense is a key ingredient in building intelligent machines that make “human-like” decisions when performing tasks — be it automatically answering natural language questions or understanding photographs and videos. So how can machines learn this common sense? While some of this knowledge is explicitly stated in human-generated text (books, articles, blogs, etc) and can be learnt by mining the web, much of this knowledge is unwritten.
While unwritten, it is not unseen! The visual world around us is full of structure bound by common sense laws depicting birds flying and balls moving after being kicked. However, machines today can not learn common sense directly from our visual world because they can not accurately perform detailed visual recognition in images and video — they can not find all the objects, recognize their interactions and describe all their properties. In this project, we propose to simplify the visual world for machines. We propose to leverage abstract scenes — cartoon scenes made from clip art by hundreds of thousands of crowd sourced humans to teach our machines common sense.
Learning common sense will make our machines more accurate, reasonable and interpretable — all imperative towards integrating artificial intelligence into our lives and society at large for:
- efficiency (personal assistants)
- healthcare (medical robots, automated care for the elderly and disabled)
- safety (autonomous driving)
- security (law enforcement, disaster recovery).
Publications
In Proceedings of the , (pp ), 02/2015
Citatations: BibTeX
Tanmay Batra, Lawrence Zitnick
In Proceedings of the International Conference on Computer Vision (ICCV), (pp 2542-2550), 05/2015
Citatations: BibTeX
In the News
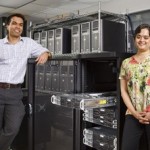
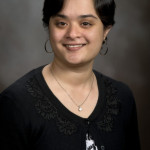
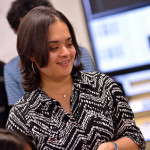
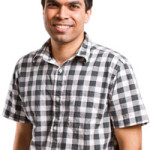