DAC Student Spotlight: Taoran Ji
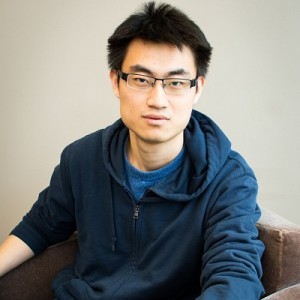
Taoran Ji, DAC Ph.D. student in the Department of Computer Science
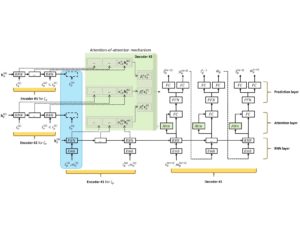
Graphic is from Ji’s paper on “Patent Citation Dynamics Modeling via Multi-Attention Recurrent Networks”
Interested in data mining and machine learning, Taoran Ji, a Ph.D. student in computer science, said he was drawn to the Discovery Analytics Center because it plays an active role in these fields.
“There are so many projects at the center that provide great opportunities to practice these techniques in real world applications,” Ji said.
Ji, advised by Chang-Tien Lu, has focused his research on a range of topics, all of which he has been able to explore by collaborating with Lu and other faculty and students at DAC. These include event detection/prediction and associated applications such as civil unrest detection, airport threat detection, transit disruption detection, and emerging science and technology prediction.
Among his published papers, two were included in proceedings at conferences held this year.
“Patent Citation Dynamics Modeling via Multi-Attention Recurrent Networks” forecasts the popularity and value of a technology of interest by developing a deep learning model to predict the number of citations that will be received by a patent or paper of interest, which can be used as an indicator of emerging technologies. Ji presented this paper at the 28th International Joint Conference on Artificial Intelligence (IJCAI 2019) in Macao, China, in August.
This research reflects Ji’s interest in discovering and forecasting emerging technologies which, he said, have great potential in the research field and can bring value to the market.
“We were able to attain access to a U.S. patent dataset, which can be viewed as the direct scientific output of science and technology activity in the industry,” said Ji. “Inspired by previous works in patent-based technology, we saw the potential value of forecasting a patent’s future citations.”
The second paper, “Feature Driven Learning Framework for Cybersecurity Event Detection,” leverages the huge volume of social media data to focus on using data mining and machine learning techniques to detect ongoing cybersecurity events and develop algorithms to automatically identify and collect online discussion and online complaints of abnormal status such as slow internet service and suspicious email logins. It was published in the IEEE/ACM International Conference on Advances in Social Networks Analysis and Mining (ASONAM 2019).
Ji’s work also includes event detection using data from social media like Twitter.
“My team and I have developed a data mining method to identify tweets denoting abnormal or suspicious events — someone brings a knife to the airport, for example — which can potentially cause security problems,” said Ji. “And in another study, we were able to detect metro service disruptions by analyzing tweets posted from the Washington, D.C., area.”
Some of this work is found in his other published papers: “Multi-Task Learning for Transit Service Disruption Detection” (ASONAM 2018); “Crowdsourcing Cybersecurity: Cyber Attack Detection using Social Media” (CIKM 2017); and “Determining Relative Airport Threats from News and Social Media” (AAAI 2017).
Ji received his master’s degree in computer science from Xidian University, China. His projected Ph.D. graduation date is 2020.