Chang-Tien Lu working with Maryland firm on advanced event prediction system to improve metro security
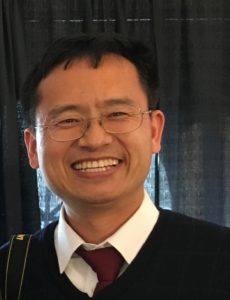
Chang-Tien Lu, a professor in the Department of Computer Science and associate director of DAC
Chang-Tien Lu, a professor in the Department of Computer Science, associate director of the Discovery Analytics Center, and a faculty member in the National Science Foundation- sponsored UrbComp program has teamed up with Schneider Electric Buildings Critical Systems, Inc. (SEBCSI) on a project entitled “Advanced Analytics for High-Performance Metro Security Monitoring,” funded by the Washington Metropolitan Area Transit Authority (WMATA)
SEBCSI, located in Columbia, Maryland, provides electronic security solutions, including security access control and closed circuit television (CCTV) for commercial and government applications.
Lu and a team of graduate students will develop an advanced spatiotemporal event detection system of several layers to deal with data preprocessing, information extraction, threat level analysis, and visualization and extract security-related information from social media contents that can help metro police improve security on trains and at metro stations.
“With the popularity of mobile devices, more public transport riders are using Twitter to report observations of potential threats, ongoing crimes, and other metro incidents. Social media serves as a good supplemental data source because tweets can cover multiple types of security issues — the most common being fight, stealth, and robbery — that are typically posted by a witness either during an ongoing crime or by the victim immediately after a crime,” said Lu.
Another advantage of social media, said Lu, is that security issue related tweets usually include station names and very accurate timestamps.
The researchers’ new system will mine collected security issues from tweet collections and visualize a crime hotspot of metro stations on a geospatial map based on location information while simultaneously representing an auto-summarized station-wise report.
“This data can help metro police make better decisions in deploying limited resources,” Lu said.
DAC students from the Department of Computer Science who are working with Lu on this project are Ph.D. student Jianfeng He and master’s students Omer Zulfiqar, Yi-Chun Chang, and Po-Han Chen.
The project builds on Lu’s previous research in intelligent transportation systems, “Steds: Social Media based Transportation Event Detection with Text Summarization,”
“A Search and Summary Application for Traffic Events Detection Based on Twitter Data,”
“Determining Relative Airport Threats from News and Social Media,” and
“TITAN: A Spatiotemporal Feature Learning Framework for Traffic Incident Duration.”