Research award aims to develop new algorithms for information extraction and understanding from scholarly literature
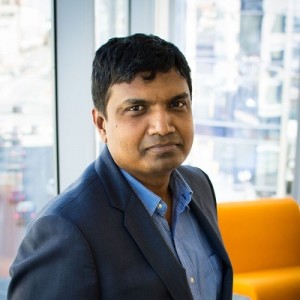
Naren Ramakrishnan, Director of DAC and Professor in the Department of Computer Science
The Discovery Analytics Center has received a research award from the Center for Security and Emerging Technology (CSET) at Georgetown University to support data-informed analysis for policymakers concerning emerging technologies and their security implications. DAC will develop methods to extract novel insights at scale from full-text analytics of publications to better understand emerging technologies and their prevalence, spatial and temporal trends, and relationships.
“Algorithmic components developed by DAC will go into a high-performance pipeline that enables inspection of extracted patterns as well as the lineage of data transformations underlying the patterns,” said Naren Ramakrishnan, the Thomas L. Phillips Professor of Engineering and DAC director, who is the principal investigator for the project.
Ramakrishnan’s team at DAC — which includes senior research associate Patrick Butler; research associate Brian Mayer; and three Ph.D. students — will develop a machine learning framework based on weak supervision to process full-text AI publications into extracted structured fields, such as information on computational platforms utilized, language and library dependencies, compute time, research methods, objective tasks, and links to source code and data resources.
The initial focus will be on arXiv as researchers evaluate and assess progress followed by extraction from China National Knowledge Infrastructure (CNKI) literature, which provides full-text articles from more than 8,000 Chinese journals covering natural sciences, engineering, technology, agriculture, medicine, and selected topics in economics and social sciences.
This project is providing DAC with the opportunity to build on its prior work in extracting information from news articles about civil unrest events. It will also be informed by DAC’s experience with automated extraction of epidemiological line lists from disease reports, which is used to develop custom word embeddings aimed at recognizing the typical language patterns in how computational details are described in the scholarly literature.
“This project brings together machine learning, computational linguistics, and human-computer interaction capabilities to extract features at scale. The information we extract will be mapped over time to help identify key trends and potential gaps that can support analysts and policy makers at the CSET,” said Ramakrishnan.
“We are looking forward to seeing how this innovative work can help inform CSET’s analysis as we strive to inform the future of AI policy,” said Dewey Murdick, director of Data Science at CSET.